AlphaDDA: strategies for adjusting the playing strength of a fully
Por um escritor misterioso
Last updated 26 novembro 2024
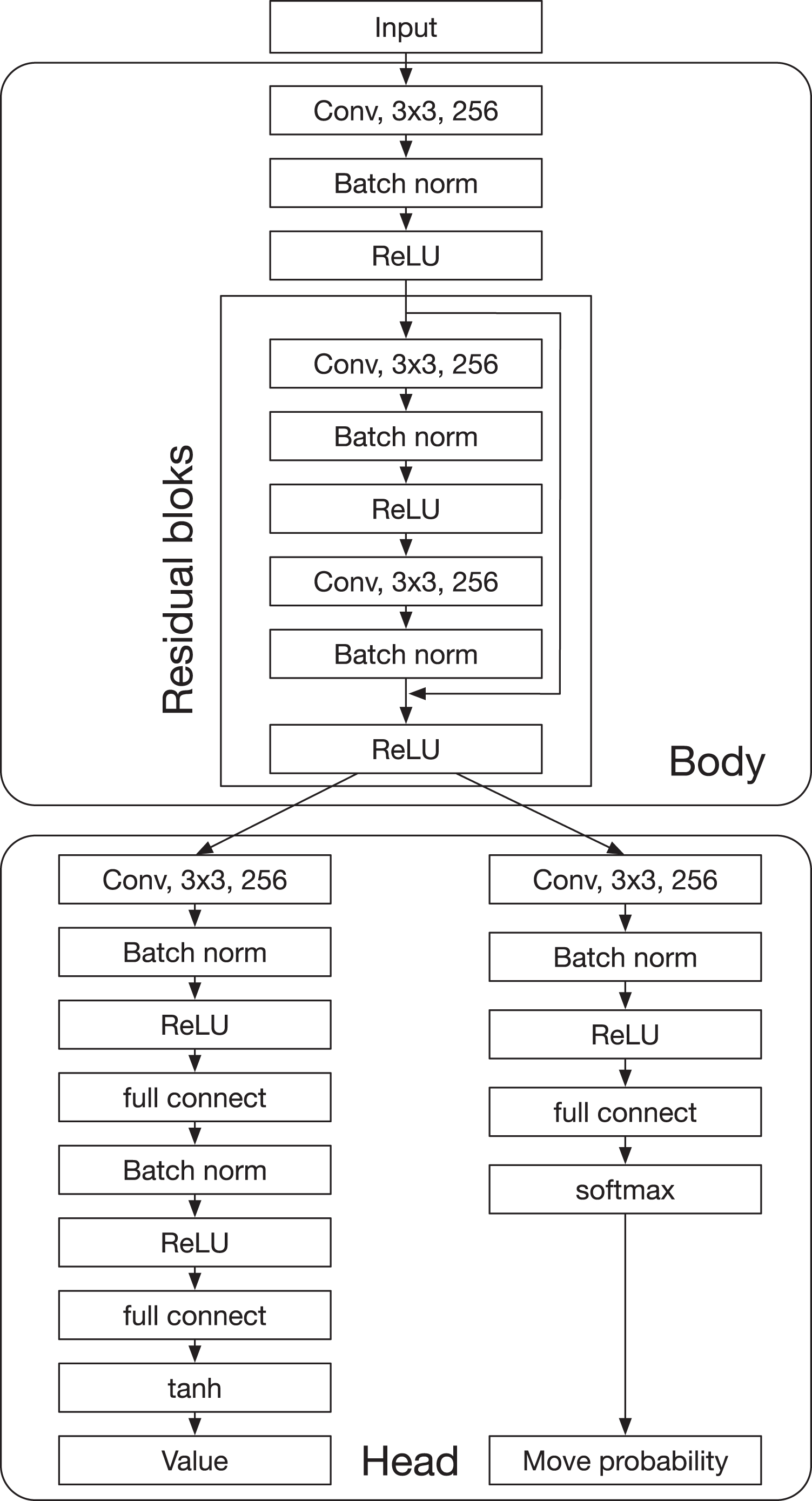
Artificial intelligence (AI) has achieved superhuman performance in board games such as Go, chess, and Othello (Reversi). In other words, the AI system surpasses the level of a strong human expert player in such games. In this context, it is difficult for a human player to enjoy playing the games with the AI. To keep human players entertained and immersed in a game, the AI is required to dynamically balance its skill with that of the human player. To address this issue, we propose AlphaDDA, an AlphaZero-based AI with dynamic difficulty adjustment (DDA). AlphaDDA consists of a deep neural network (DNN) and a Monte Carlo tree search, as in AlphaZero. AlphaDDA learns and plays a game the same way as AlphaZero, but can change its skills. AlphaDDA estimates the value of the game state from only the board state using the DNN. AlphaDDA changes a parameter dominantly controlling its skills according to the estimated value. Consequently, AlphaDDA adjusts its skills according to a game state. AlphaDDA can adjust its skill using only the state of a game without any prior knowledge regarding an opponent. In this study, AlphaDDA plays Connect4, Othello, and 6x6 Othello with other AI agents. Other AI agents are AlphaZero, Monte Carlo tree search, the minimax algorithm, and a random player. This study shows that AlphaDDA can balance its skill with that of the other AI agents, except for a random player. AlphaDDA can weaken itself according to the estimated value. However, AlphaDDA beats the random player because AlphaDDA is stronger than a random player even if AlphaDDA weakens itself to the limit. The DDA ability of AlphaDDA is based on an accurate estimation of the value from the state of a game. We believe that the AlphaDDA approach for DDA can be used for any game AI system if the DNN can accurately estimate the value of the game state and we know a parameter controlling the skills of the AI system.
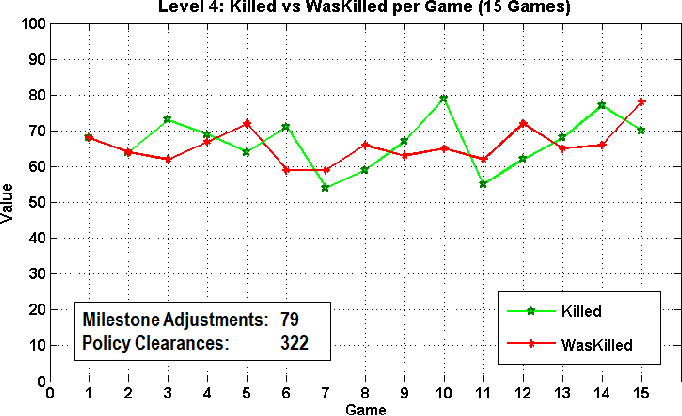
PDF] Skilled Experience Catalogue: A Skill-Balancing Mechanism for Non- Player Characters using Reinforcement Learning
An overview of Skilled Experience Catalogue.
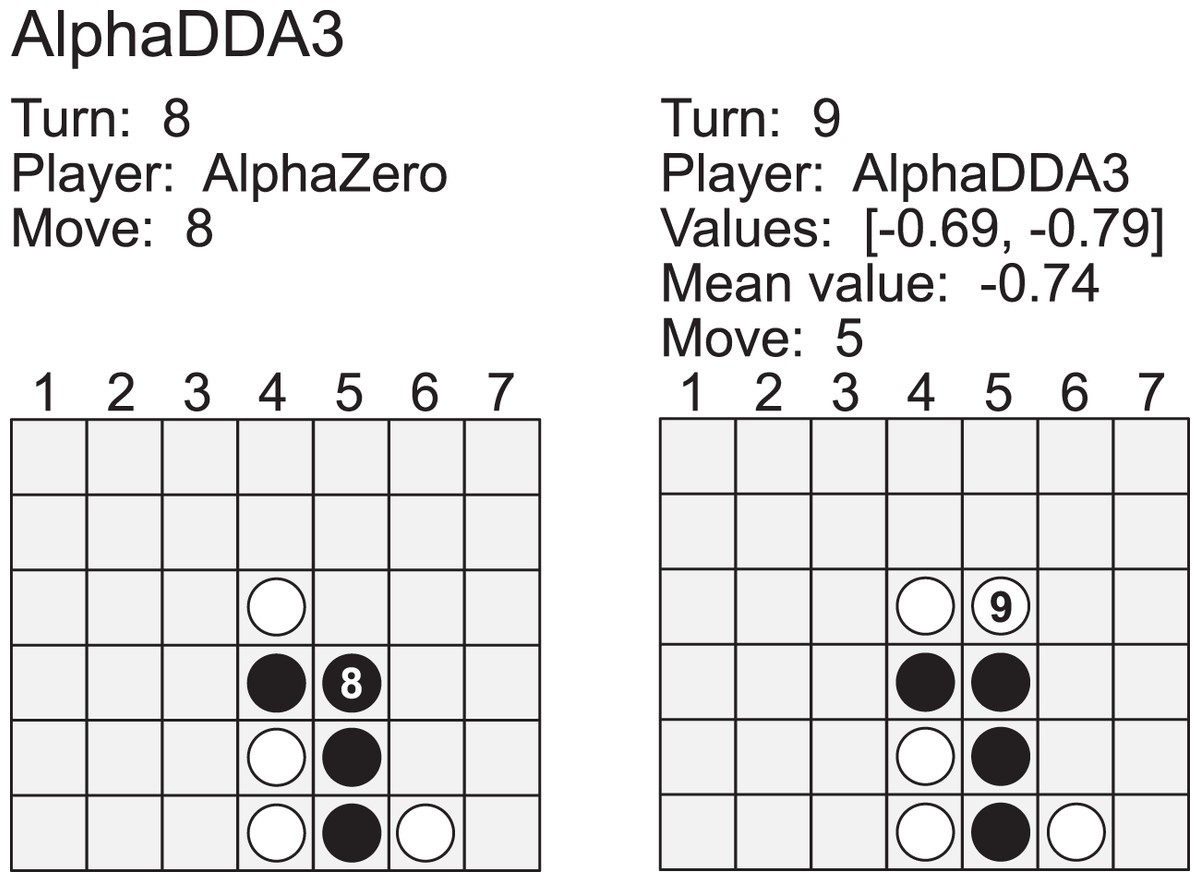
AlphaDDA: strategies for adjusting the playing strength of a fully trained AlphaZero system to a suitable human training partner [PeerJ]
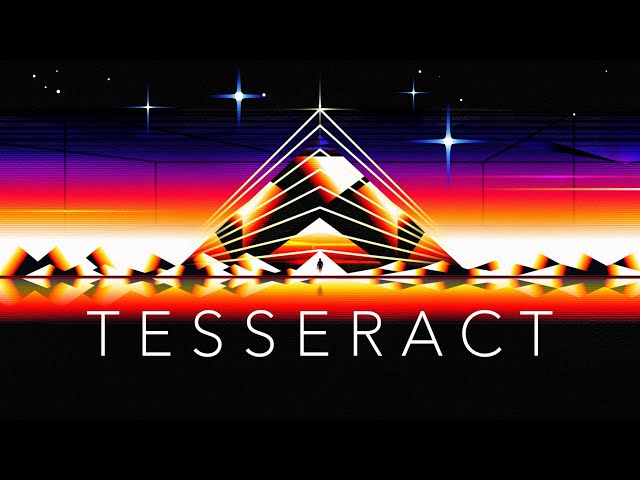
Game Changer: AlphaZero's Groundbreaking Chess Strategies and the Promise of AI

arxiv-sanity
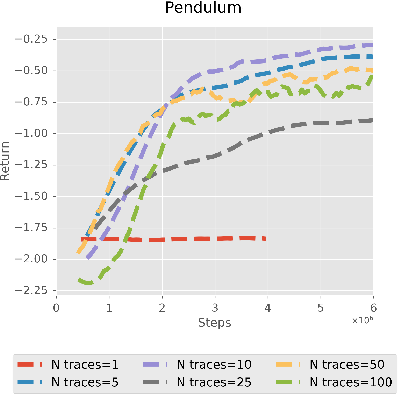
PDF] A0C: Alpha Zero in Continuous Action Space
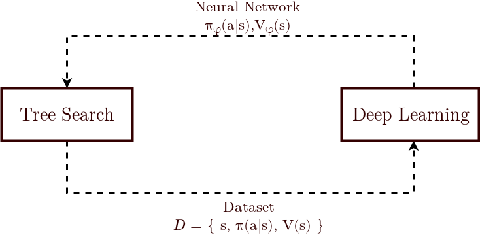
PDF] A0C: Alpha Zero in Continuous Action Space
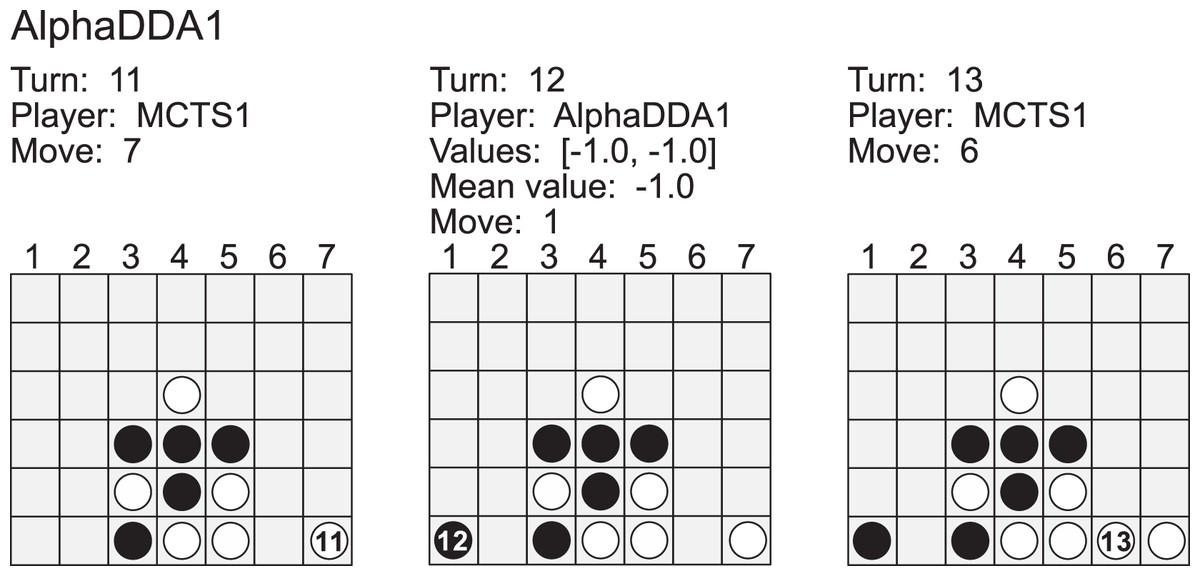
AlphaDDA: strategies for adjusting the playing strength of a fully trained AlphaZero system to a suitable human training partner [PeerJ]
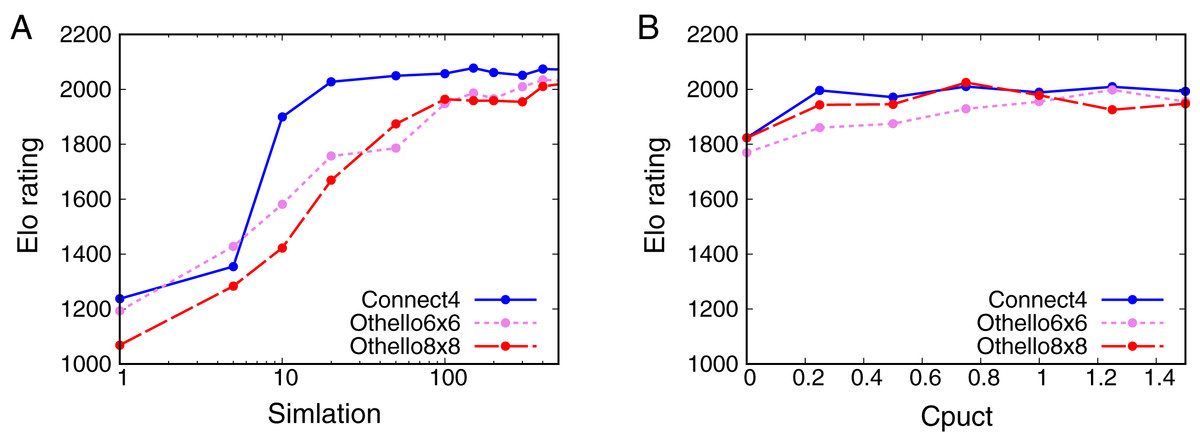
AlphaDDA: strategies for adjusting the playing strength of a fully trained AlphaZero system to a suitable human training partner [PeerJ]
Recomendado para você
-
Google AI Achieves Alien Superhuman Mastery of Chess and Go in Mere Hours - The New Stack26 novembro 2024
-
Multiplayer AlphaZero – arXiv Vanity26 novembro 2024
-
Home · AlphaZero26 novembro 2024
-
GitHub - peldszus/alpha-zero-general-lib: An implementation of the26 novembro 2024
-
GitHub - aqtq314/AlphaZero: A Keras implementation of Google's26 novembro 2024
-
GitHub - Yangyangii/AlphaZero-connect6: DeepMind AlphaZero for26 novembro 2024
-
GitHub - CogitoNTNU/AlphaZero: An implementation of AlphaZero26 novembro 2024
-
redis - golang Package Health Analysis26 novembro 2024
-
Alpha Zero General playing Tic Tac Toe in p5 using tf.js — J26 novembro 2024
-
AlphaZero and beyond: Polygames26 novembro 2024
você pode gostar
-
Macacão Poppy Playtime Huggy Wuggys Monstro, Fantasia de Halloween26 novembro 2024
-
Gadgets 360 on X: Steam's Spring Sale Includes God of War, FIFA26 novembro 2024
-
ghost do outro lado da vida filmes antigos26 novembro 2024
-
Um cão branco com uma mancha preta em um olho em um parque, pastor suíço branco misturado com ponteiro inglês26 novembro 2024
-
Peppa Pig - Casinha Candy - Festa E Decoração em Promoção na Americanas26 novembro 2024
-
Ew Games Bauru SP26 novembro 2024
-
Similar to Spider-Man Remastered, Will There Be a Stan Lee Easter26 novembro 2024
-
Senpai ga Uzai Kouhai no Hanashi – 05 – Random Curiosity26 novembro 2024
-
Controle Joystick Com Fio Compatível Xbox One Pc Notebook - N-126 novembro 2024
-
Zombicide 2nd Edition — The Village Geek26 novembro 2024