Efficient and Accurate Candidate Generation for Grasp Pose
Por um escritor misterioso
Last updated 22 março 2025

Recently, a number of grasp detection methods have been proposed that can be used to localize robotic grasp configurations directly from sensor data without estimating object pose. The underlying idea is to treat grasp perception analogously to object detection in computer vision. These methods take as input a noisy and partially occluded RGBD image or point cloud and produce as output pose estimates of viable grasps, without assuming a known CAD model of the object. Although these methods generalize grasp knowledge to new objects well, they have not yet been demonstrated to be reliable enough for wide use. Many grasp detection methods achieve grasp success rates (grasp successes as a fraction of the total number of grasp attempts) between 75% and 95% for novel objects presented in isolation or in light clutter. Not only are these success rates too low for practical grasping applications, but the light clutter scenarios that are evaluated often do not reflect the realities of real world grasping. This paper proposes a number of innovations that together result in a significant improvement in grasp detection performance. The specific improvement in performance due to each of our contributions is quantitatively measured either in simulation or on robotic hardware. Ultimately, we report a series of robotic experiments that average a 93% end-to-end grasp success rate for novel objects presented in dense clutter.

Robust grasping across diverse sensor qualities: The GraspNet
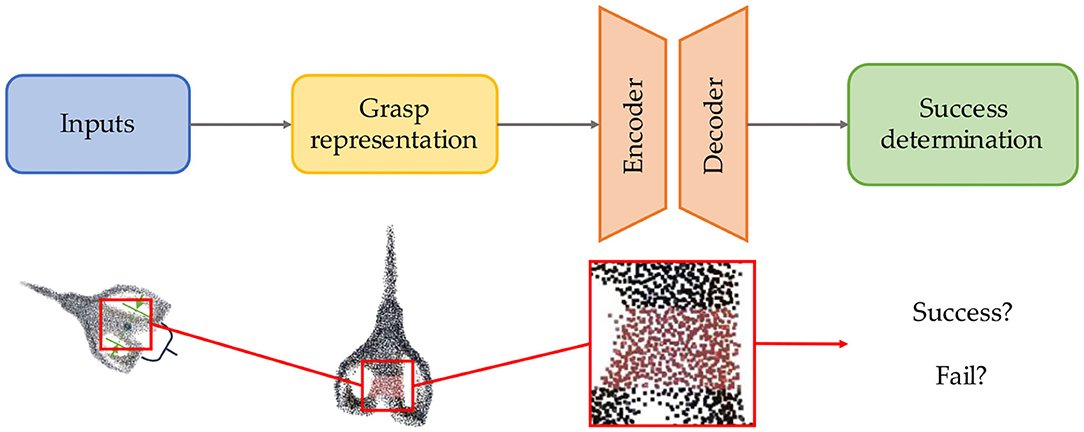
Frontiers Robotics Dexterous Grasping: The Methods Based on
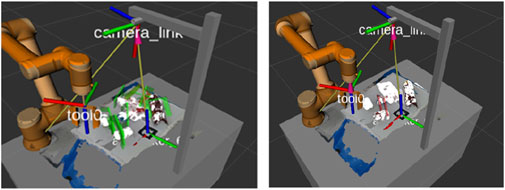
Frontiers Learning-based robotic grasping: A review
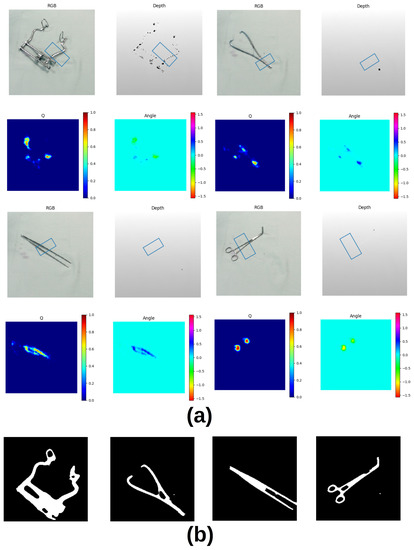
Robotics, Free Full-Text

Left: A grasp g is defined by its Cartesian position (x, y, z
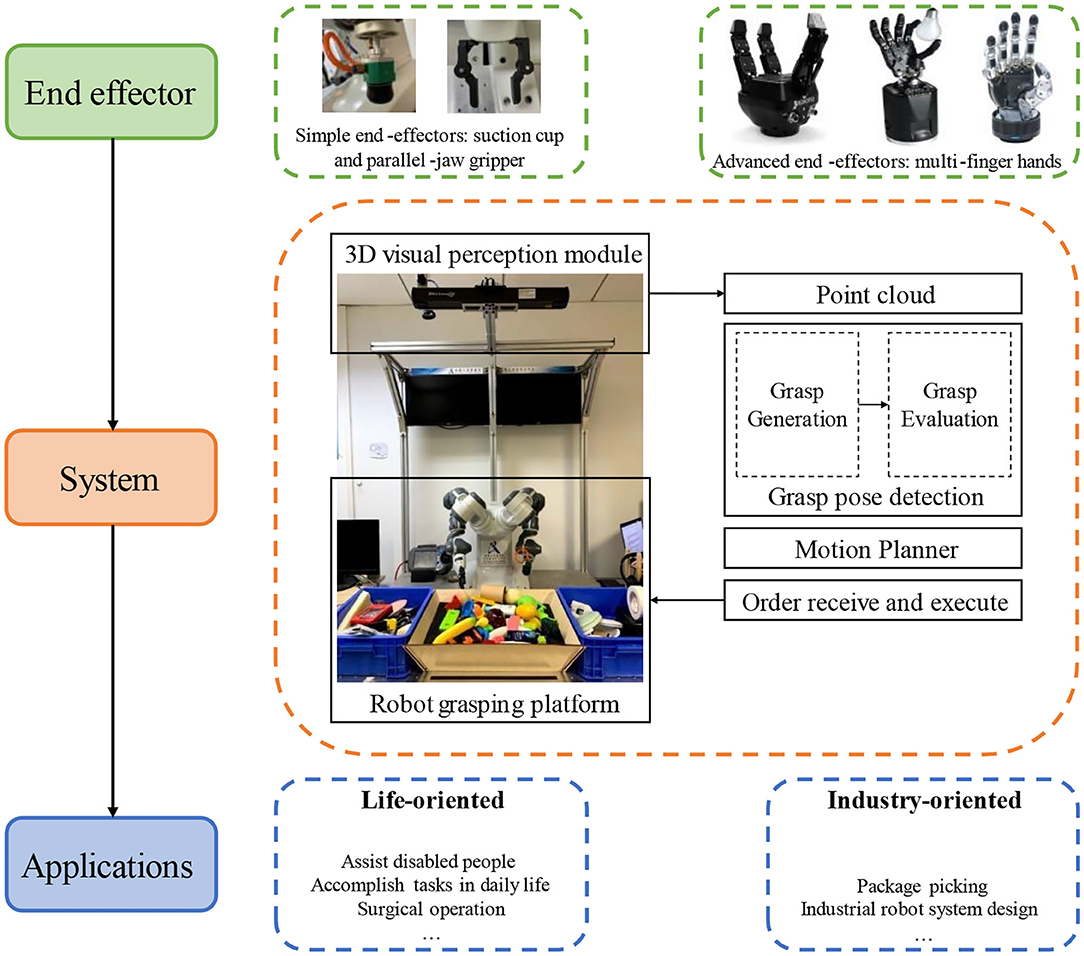
Frontiers Robotics Dexterous Grasping: The Methods Based on

Grasp Pose Detection in Point Clouds - Andreas ten Pas, Marcus

3D Grasp Pose Generation from 2D Anchors and Local Surface

Grasp pose representation in the camera frame
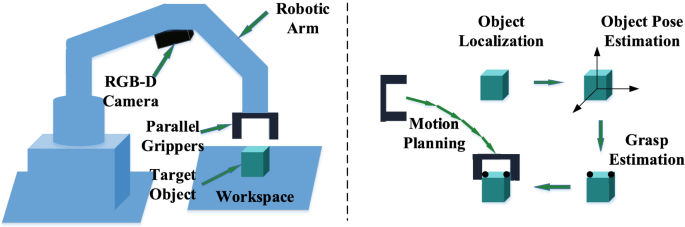
Vision-based robotic grasping from object localization, object
Recomendado para você
-
vagas on X: #DICARAPIDA 🚗 💨 Candidate-se em oportunidades22 março 2025
-
La France au Mozambique on X: 🎓📚🇫🇷⚠️Candidate-se a uma22 março 2025
-
Nossa história - Se Candidate, Mulher!22 março 2025
-
Dagoberto Loiacone on LinkedIn: Candidate-se no link22 março 2025
-
Driving Urban Transitions: Candidate-se22 março 2025
-
Se Candidate, Mulher!22 março 2025
-
Adjustable Universal Motorcycle Phone Mount Candidate-se A Loncin22 março 2025
-
Candidate-se agora - Kemira22 março 2025
-
Novo Bauhaus Europeu Candidate-se até 28 de fevereiro aos22 março 2025
-
2 páginas de carreira para seguir nas redes sociais22 março 2025
você pode gostar
-
In Another World With My Smartphone season 2 premieres in April - Niche Gamer22 março 2025
-
BG 1 [Naruto Mobile] by Maxiuchiha22 on DeviantArt22 março 2025
-
Roleplay as a landlord in The Sims 4's upcoming real-life22 março 2025
-
Resident Evil Code Veronica Remake is Happening next Year in 202422 março 2025
-
Chess Openings – Chess Academy of Denver22 março 2025
-
2011 Mavs Playoffs Rewind: Mavericks rise to the challenge; beat Blazers 93-82 in redeeming game five - Dallas Sports Fanatic22 março 2025
-
Lutador Super Dino - Jogo Online - Joga Agora22 março 2025
-
Red Pyramid Thing Statue by First 4 Figures22 março 2025
-
Enhanced Predator (The Predator) vs Alien Queen: Who wins? : r/LV42622 março 2025
-
História Death Parade - Juízes não podem sentir emoções - História22 março 2025